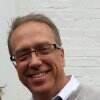
Accelerating Innovation in the Analytic Ecosystem: Simplicity
In part of this series on reducing conflict between business & IT to accelerate innovation, we focus on enabling simplicity for compute, storage and connectivity.

In the series on accelerating innovation in the analytics ecosystem, we are focused on understanding the larger problem domains from both an IT and business perspective. In this article we will focus on Simplicity.
As stated originally, recognizing the conflict is the first step in understanding the broader problem. No one is at fault and both are only focusing on meeting their objectives. It can appear we are at an impasse, but there is a solution.
The goal is a win-win outcome enabled by Business and IT simultaneously achieving their objectives with the least friction possible. We will continue to leverage the analytics capability framework in Figure 1 to focus on the different needs of the business and IT, and provide recommendations for meeting those needs.
The framework below depicts 3 foundational capabilities required for success in a modern analytic architecture. These three capabilities are:
- Flexibility – The ability to choose the most appropriate software resources, e.g. tools, languages and libraries, to accelerate the user’s time to insight and minimize operationalization efforts.
- Simplicity – The ability to quickly provision and decommission analytic resources, e.g. compute, storage and network, in a simplified, manageable and cost-effective manner for business user and IT.
- Accessibility – The ability to efficiently find, secure and govern information and analytics within the entire analytic ecosystem without slowing down the business users or jeopardizing production.

Figure 1 – Analytics Capability Framework
Enabling Simplicity for Compute, Storage and Connectivity Without Losing Control
Modern analytic ecosystems include capabilities that didn’t exist a few years ago. These new flexible capabilities increase complexity. Part of the increase in complexity can be attributed to characteristics related to data, performance, storage, compute, and connectivity. Increased desire for more analytics combined with increased data volumes, velocity and variety have increased the need for data stores with varying performance and cost characteristics. Computation needs for more complex enterprise wide analytics have forced the need for more scalable, powerful computing resources with variable performance and cost.
Business users need compute resources more powerful than their desktops which leverage massively parallel computing, possibly GPU computing, with large amounts of memory and high bandwidth I/O. They need the ability to choose the right compute engine and data storage for their analytic problem. They may need these resources today, but possibly not tomorrow. Additionally, they need workloads executed on computing resources which are dynamically allocated at runtimes based on demand which could vary greatly.
The users need the ability to easily access and store potentially large volumes of data. To perform analytics, they need data from multiple systems in various formats residing in S3 compatible object stores or distributed file stores, e.g. HDFS. They need access to quality production data along with the ability to bring their own data. Quite often users need to combine data from multiple data stores and join it with data they’ve loaded into their discovery sandbox. Having multiple analysts manually moving data from multiple production platforms into discovery sandbox is time consuming, wastes resources, encourages data sprawl and lowers data quality over time via data drift. Users need to be able to query data easily and seamlessly without having to manually wrangle data from disparate data sources to execute analytics.
The IT team needs to manage analytic resources used in production and discovery environments. IT must ensure that production workloads meet SLAs, but also need to ensure that analytic discovery resources are available, accessible and secured. For IT, it is critical that IT resources provisioned for analytic sandboxes are sanctioned, flexible and well managed.
Business Needs:
- Access to storage and compute resources to support business analytics needs
- Simplified provisioning process to obtain resources for analytic exploration and innovation
- Easy access to data in multiple data stores via virtualization to reduce the need for data replication
- Simplified analytic entry point
IT Needs:
- Standardized provisioning of sanctioned resources for discovery analytics to ensure supportability and speed time to value
- Ensure production SLAs are being met
- Enabling virtualization capabilities to simplify data access and analytic processing over a high-speed fabric
- Optimizing and rationalizing resources for cost efficiencies
- Manage / monitor production & discovery resource usage
Recommendations:
Recommendations for enabling simplicity for compute, storage and connectivity:
IT Focused
- Provide an analytics discovery/innovation platform with adequate compute, storage and connectivity to support the analytic needs of the business. Leverage new technology such as object stores which provide very low-cost storage with reasonable data transfer rates at higher latencies which is acceptable for most analytics running against large data sets.
- Promote an environment which minimizes the friction of moving analytics from exploration into production.
- Create analytic ops frameworks and processes which enable easily promotion of new data and analytics into production with the proper governance to ensure ongoing quality in the data and analytics.
- Proactively work with the business on evaluating new tools and technologies which meet their needs, are approved and funded, and rationalized into the analytic ecosystem.
- Establish simple auto-provisioning capabilities for users to establish sandbox resources.
- Instantiate sanctioned resources which are powerful enough to meet user’s analytic needs with enough monitoring to be efficiently managed.
- Provide provisioning defaults by persona, but allow for exceptions.
- Provide a high-speed data fabric between data storage platforms which reduce data transfer latency and minimize the need for data replication.
- Establish data virtualization capabilities and policies which simplify access to data in multiple data stores.
- Automate the creation of foreign tables to support simple SQL based access to data across platforms.
- Balance IT needs of performance, flexibility and total-cost-of-ownership for the analytic ecosystem, with the business needs for discovery and innovation.
- Implement workload management capabilities which can ensure production can meet its SLAs while enabling discovery environments to directly access production data.
Business Focused
- Utilize IT sanctioned environments to minimize effort and time to move discovery analytics into production.
- Leverage IT provided auto-provisioning process to speed access to analytic environments.
- Leverage data virtualization to join data on-demand via SQL from multiple data stores instead of creating load jobs, minimizing data replication, improving data quality and maximizing speed to insight.
- Proactively work with IT on new technologies which address business gaps.
- Focus on time to insight vs performance while in discovery.
Simplicity enables users to quickly provision their own analytic sandboxes to focus on analytics for solving their business problems. When IT provides the simple provision capabilities to the user community, it can ensure the resources can be supported, monitored and managed to provide visibility into the data being loaded and the analytics processes being executed. The users have great freedom of the various types of resources they can leverage, and IT has visibility of the resources allocated and being utilized.
Stay in the know
Subscribe to get weekly insights delivered to your inbox.